Introduction

Explainable AI, or XAI, as the name suggests, is a tool that can be used to analyze and explain the works of an AI model. It is used to describe an AI model so that we know exactly how the learning and predictions happen. Being able to transparently see how an AI model works is crucial to building trust and confidence within organizations.
With explainable AI, organizations can have a better experience training and fine-tuning the model, since now they know exactly why they receive the output they receive. As this field becomes more sophisticated and complicated, it becomes harder for the developer to understand the AI, and why it does what it does. All we know is about the input we give in, and the output we receive, everything between the input and output is invisible, and that’s not good.
Nowadays, many companies that use AI, are not sure about exactly how their AI lands on a prediction. There is no way for them to explain how their algorithm got them to that particular result. With the rise of explainable AI, we can now have better control over the AI products that we build, along with making sure about the prediction quality and accuracy.
If you are new to the industry, the need for XAI might not be straightforwardly apparent, so let’s look into why being able to read an AI model’s working is a necessity.
The Black Box Problem and the Need for XAI
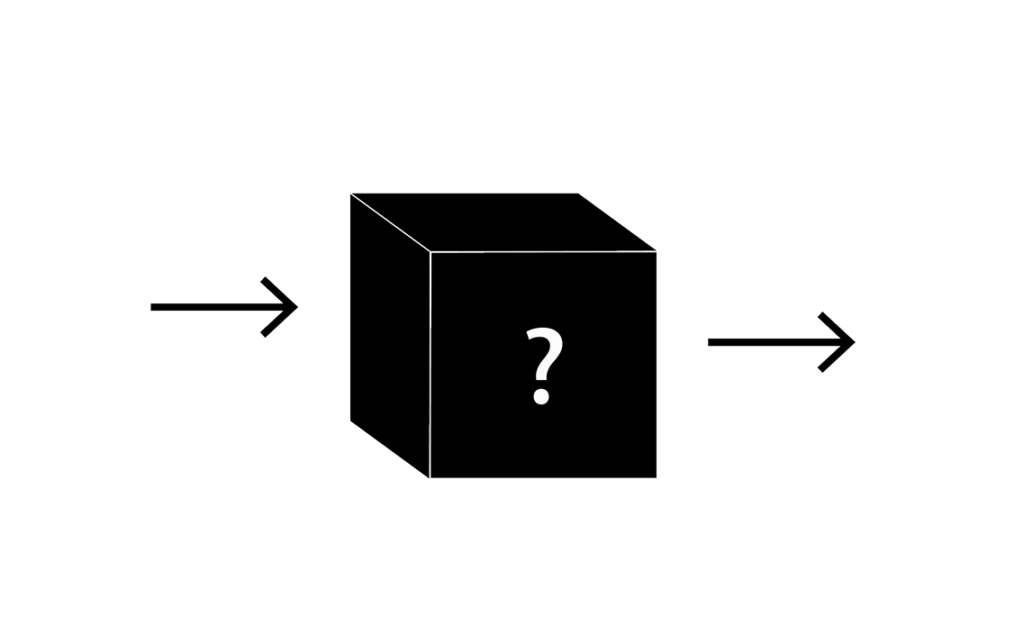
Nowadays, the AI Models we build a super powerful, but concerns arise when these models act as “Black Boxes”. A black box model is a model whose operations are inconceivable for humans. As we discussed in the previous section, when we work with these kinds of models, all we can understand are the input data that went in, and the output predictions or results, how the model got to the decision is unexplainable.
Now this can pose serious concerns when we deploy the model into industries like medicine, law, or finance. Imagine the model recommends a medical treatment, but nobody knows how it came to that decision. Imagine another model giving you financial advice, but you cannot explain how and why it got to the decision. A black box model can never be trusted in industries where many things can go wrong if the ai makes a wrong decision.
High odds of improper debugging can ruin these models, along with trust issues. When you don’t know how an AI model works before it gets to a decision, you can’t really say where you are going wrong, if you are going wrong. When there is bias in the data that’s been provided, a developer can easily spot it when the model is transparent. This is nearly impossible when the model is all opaque and black.
How can XAI be Used?
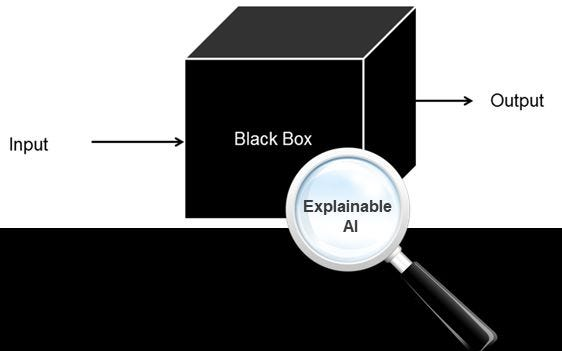
XAI offers several implementation options for your model, though the most suitable approach depends heavily on your model’s type and complexity.
- Feature Importance: Every time you use a dataset to train your AI, you can always see how some features are more important than others. Say you build a model to predict housing prices. Features like the land size, number of bedrooms, age, and location might matter more than other minor details like distance to the airport, or nearest school. Feature importance can let you know exactly what features would affect the model predictions more than the others.
- Visualize Decision Trees: Decision Trees are a great way to visualize models. In a decision tree, there are just a bunch of questions asked by the model to predict a value. In simpler models, developers can take advantage of visualization in decision tree predictions and explain how the AI model comes to a particular decision.
- Counterfactual Explanations: This method involved exploring cases that could have happened. In other words, instead of just accepting a result from the AI model, the developer can try exploring possibilities of how a small change in the data can change the prediction. By doing this, you can not only see how the row value in each feature can affect the prediction but also help you understand alternative scenarios.
Other Methods
- Model Agnostic Methods: This method can be used on any kind of model where you can explain the model just by using the input and output values. Some of the most used Model Agnostic Methods are Local Interpretable Model-Agnostic Explanations (LIME) and SHapley Additive exPlanations (SHAP).
- Gradient Based Methods: This method, is specifically applicable for deep learning models with insane training. It involves looking at the rate of changes (gradients) in the predictions based on the inputs and come to a conclusion about how the input features affect the predictions.
- Rule Extraction: This technique involves creating a set of rules that mimic the model in such a way that it is human-interpretable. This can be helpful in providing model insights and a clear understanding of how it works.
Benefits of Explainable AI
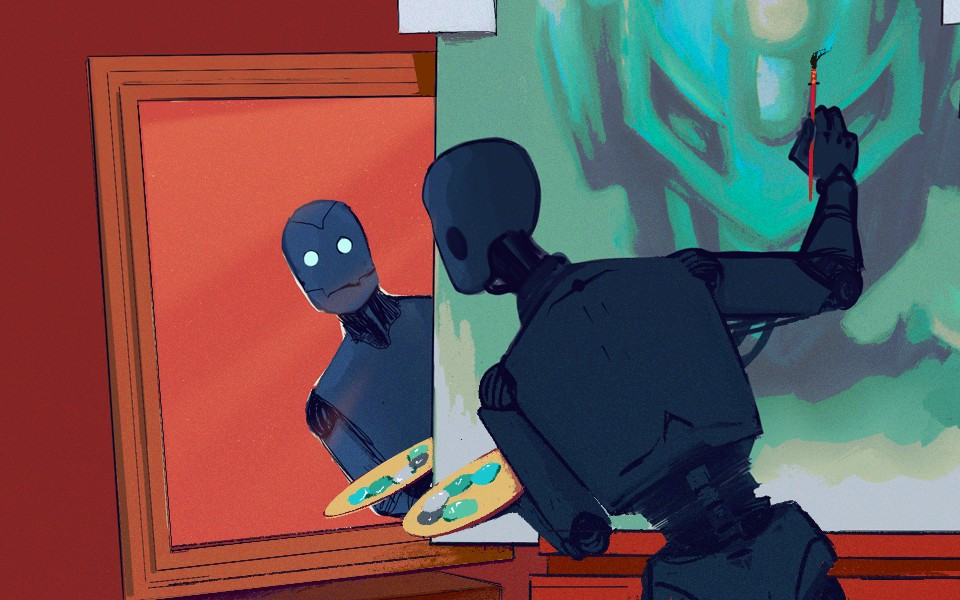
By now it should be obvious that explainable AI can not only be beneficial to an AI model but also ensure efficiency, authenticity, and transparency when you deploy it.
By understanding the model, developers can ensure privacy and fairness in the model, which allows them to handle risks and deploy a responsible model. Deploying a model with explainable AI in an industry ensures compliance with regulations. This is because the model becomes a white box, where developers and the team fully understand its workings, unlike a mysterious black box model.
Additionally, building a model that is transparent to everyone, would allow you to create a very user-interactive product. This allows the users to tweak the input features in a way that allows them to understand why the model gets to a certain output. This can also let the users to better understand the capabilities and limitations of the model.
White box models enable human evaluation of AI outputs, which significantly aids in model improvement. Human involvement with the model would result in better decision-making, and this requires using explainable AI on complicated models.
The Future of Explainable AI (XAI)
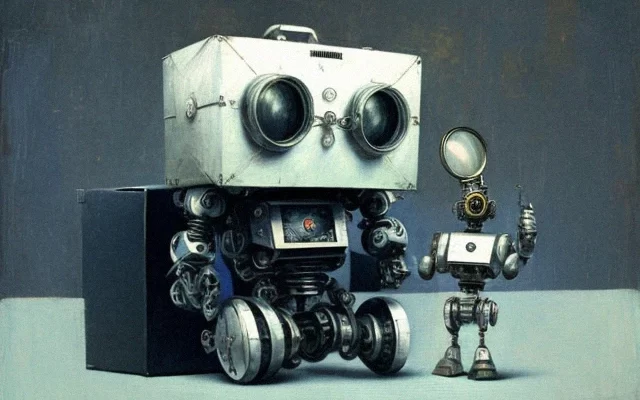
As AI models continue to grow, and become a part of critical decisions, public trust, and regulations become a primary requirement for the adoption of a model. As we have discussed in the previous sections, the role of Explainable AI is exactly to accomplish this. Researchers will develop new frameworks to explain models that are more complex and use Deep Learning.
As XAI continues to develop, human feedback in models becomes easier and flawless, potentially correcting biases within the model predictions. Moreover, this technology will advance to a point where it starts adapting to the user’s background and experience in the field to explain everything perfectly in such a way that it is easier for everybody to understand the workings and tune the models accordingly.
At one point, XAIs will not only provide us with insights regarding the model but will also suggest corrections that we as humans can make to the model to get the best results. Overall, the developments in the field would let developers build prediction models and fine-tune them with minimal effort.
Towards a More Transparent AI Future
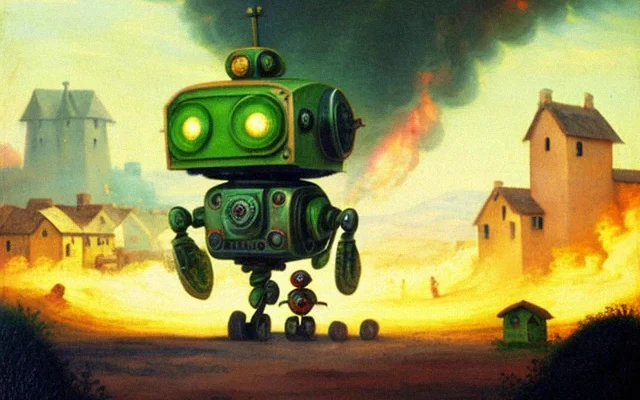
Building a more transparent AI future is necessary not only to ensure fairness and ethics in the usage of AI but also to practice complex models in industries where mistakes can cost a lot. Additionally, at one point building AI models that can make good predictions over sophisticated data would require transparency to make sure the model performs well. The increasing complexity of AI models is driving the need for XAIs (Explainable Artificial Intelligence).
To sum it up, we use XAI to describe an AI Model and to understand how the model arrived at a certain prediction. The need for XAI arises due to the black box problem, where black box models are models where the developers have no idea how the model works on the inside. We should avoid these kinds of models because they can erode trust among users. The opposite of this is the white box models – models that are transparent, where the developers and users know exactly what’s going on and why the model works the way it works.
Pretty element of content. I just stumbled upon your weblog and in accession capital to claim that I acquire in fact enjoyed account your weblog posts. Any way I’ll be subscribing in your feeds or even I success you get admission to consistently quickly.
Awesome page with genuinely good material for readers wanting to gain some useful insights on that topic! But if you want to learn more, check out YH9 about Entrepreneurs. Keep up the great work!
Hi there, I simply couldn’t leave your website without saying that I appreciate the information you supply to your visitors. Here’s mine QH8 and I cover the same topic you might want to get some insights about Thai-Massage.
You’ve written terrific content on this topic, which goes to show how knowledgable you are on this subject. I happen to cover about Social Media Marketing on my personal blog Article Sphere and would appreciate some feedback. Thank you and keep posting good stuff!
It appears that you know a lot about this topic. I expect to learn more from your upcoming updates. Of course, you are very much welcomed to my website Articleworld about PR Marketing.
This was a very good post. Check out my web page Articlecity for additional views concerning about Data Mining.
This was a very good post. Check out my web page Autoprofi for additional views concerning about Used Car Purchase.
Your posts in this blog really shine! Glad to gain some new insights, which I happen to also cover on my page. Feel free to visit my webpage ArticleHome about Data Mining and any tip from you will be much apreciated.
Awesome page with genuinely good material for readers wanting to gain some useful insights on that topic! But if you want to learn more, check out Seoranko about Blogging. Keep up the great work!
As someone still navigating this field, I find your posts really helpful. My site is Webemail24 and I’d be happy to have some experts about SEO like you check it and provide some feedback.